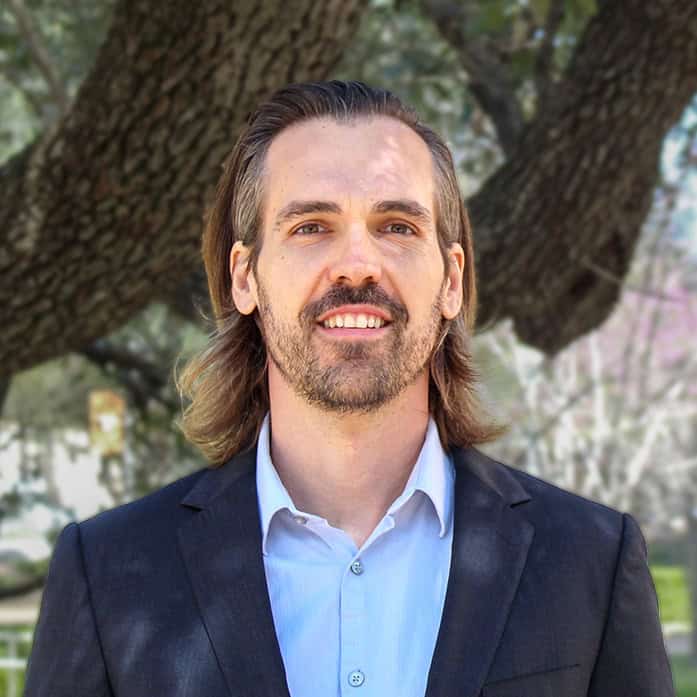
Brad Knox
Why do you care about AI Existential Safety?
My concern for AI existential safety is based on the immense impact AI will haveāboth positive and negative. From my early work pioneering reinforcement learning from human feedback (RLHF), I’ve mostly focused on how humans can communicate what they want to AI. This is not merely a technical challenge but a moral imperative. In my research, I’ve seen firsthand how misaligned AI poses significant risks and fear the consequences of misalignment with the ever-increasing agency and capabilities of AI. Examples like reward functions in autonomous driving that misinterpret human preferences illustrate the gravity of these risks. My transition from industry to academia, specifically to focus on AI alignment, further demonstrates my concern for ensuring AI advancements contribute positively to society. I believe that without meticulous research and thoughtful consideration in designing and deploying AI systems, we risk unforeseen consequences that could have far-reaching impacts. Consequently, my dedication to AI existential safety research comes from a desire to prevent potential catastrophic outcomes and to steer AI development towards beneficial and safe applications for humanity.
Please give at least one example of your research interests related to AI existential safety:
The majority of my research career has focused on AI alignment, which appears to be critical for reducing x-risk from AI. My dissertation at UT Austin pioneered the reinforcement learning from human feedback (RLHF) approach, now a key training step for large language models (LLMs). I concluded my dissertation defense by highlighting RLHF’s potential to empower humans in teaching AI to align with their interests (video). During a postdoc at MIT, I led the first course worldwide on interactive machine learning and co-authored the field’s most cited overview. At this stage, while not explicitly focused on existential risk, I was already contemplating human-AI alignment.
Later, I returned to academia as a labās co-lead. I was hired to work on reinforcement learning (RL) for autonomous driving but was drawn to a niche: how the AI could know humans’ driving preferences and how existing research communicates such preferences. In RL, these preferences are communicated with reward functions.
So began my current alignment research. The results so far are listed here. I have focused on human-centered questions critical to alignment but too interdisciplinary for most technical alignment researchers. I have considered how reward functions could be inferred from implicit human feedback such as facial expressions (website). I have identified issues resulting from the ad hoc trial-and-error reward function design used by most RL experts (website). And I have found that published reward functions for autonomous driving can catastrophically misspecify human preferences by ranking a policy that crashes 4000 times more often than a drunk US teenager over a policy that safely chooses not to drive (website). I also have published three papers that begin with questioning the previously unexamined assumption within contemporary RLHF of what drives humans to give preferences (see the final three papers on this page). This research has all been published in or accepted to top AI venues.
While most computer science researchers make mathematically convenient assumptions about humans without questioning them, which can provide a misaligned foundation upon which they derive algorithms that result in misaligned AI. Instead, I take an interdisciplinary approachāblending computer science with psychology and economics, as demonstrated by the projects aboveāwhich positions me to provide unique insights at the intersection of AI and the human stakeholders its decisions affect, insights that are complementary to and serve as a check for pure computer science research on alignment.